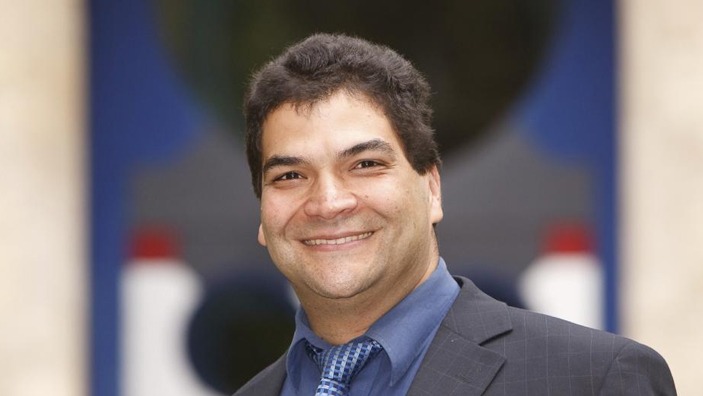
The swift rise of generative artificial intelligence (AI), in which machine learning goes beyond drawing conclusions from large datasets to producing new content from existing data, has led to plenty of conversation in recent years – and particularly since the spectacular popularity of ChatGPT since it became available in late 2022.
These concerns have led to optimism about the potential benefits of AI in medicine and other fields, owing to the ability to tap into a vast array of knowledge. Yet it has also led to what Michael Barrett, Professor of Information Systems & Innovation Studies at Cambridge Judge Business School, believes is a polarised debate that includes a range of “utopian and dystopian imaginations”.
This article summarises an editorial in the journal ‘Information and Organization’, co-authored by Professor Michael Barrett of Cambridge Judge Business School, that outlines some of those risks.
Why policymakers must think far ahead on AI risks
To better focus the debate on practical implications and solutions, Michael and academic colleagues have published an editorial in the journal ‘Information and Organization’, where Michael is Editor in Chief, in which they focus on practical approaches to address some of the downsides of AI. These include algorithmic bias that benefits those with access to training data, the ‘colonisation’ of data through the offshoring of algorithm creation, and the risk that certain communities and interests could be marginalised through the widespread use of AI.
“We propose a relational risk perspective as a useful lens in studying the dark side of AI,” says the editorial. “Our goal is to build prescient knowledge regarding how AI and its emerging use may shape possible futures in order to sharpen our critical thinking around the potential risk of emerging data-driven technologies.”
The editorial – entitled “Risk and the future of AI: Algorithmic bias, data colonialism, and marginalization” – is co-authored by Dr Anmol Arora of the School of Clinical Medicine at the University of Cambridge, Professor Michael Barrett of Cambridge Judge Business School, Dr Edwin Lee of the Centre for Digital Innovation (CDI) at Cambridge Judge, Professor Eivor Oborn of Warwick Business School and a Fellow at CDI, and Karl Prince of CDI.
Nine findings on the risks and future of AI
Professor Michael Barrett discusses some of the points raised in the editorial, along with broader perspectives about AI and 21st century society.
1
Algorithmic bias has huge potential for diversity, inclusion and marginalisation
Algorithmic bias has huge potential for diversity, inclusion and marginalisation in the workplace and beyond.
We talk about data ‘colonisation’ with regards to potential harm suffered by workers in the Global South who clean up data and develop algorithms for the benefit of those using algorithms in the Global North. Data is central to AI technologies, so the access and development of this data can lead to increased data inequality, and this is a big risk.
2
We need to act now to prevent potential risks from AI, not simply clean up later
We need to consider consequences in advance, not after the fact, because by acting in advance we may be able to influence the design and eventual outcomes of AI-related technologies.
3
The risks of AI require a new approach
The risks of AI, especially at the frontier of development, require a new way of thinking
A relational view of risk is needed in envisioning the future of AI as an emerging technology, and in conceptualising risk as a duality that integrates both benefit and harm.
4
Health data is in danger due to algorithmic bias
An algorithm may perform really badly on a particular population or other group if the algorithm is not exposed to data from that population while the algorithm is being developed and people are trained on it – and data from marginalised patient groups is often underrepresented. So this leads to a vicious cycle in which algorithms don’t reflect healthcare data of certain groups, and those algorithms then deepen health inequalities affecting those communities. Synthetic data, although it has many problems of its own, has been used to counter algorithmic bias in some healthcare situations.
5
AI presents new risks for offshoring
While offshoring has existed in the data world for decades now, we see a particular new risk with regards to AI.
The term ‘data colonialism’ reflects a parallel with historical colonialism, but data has characteristics that go beyond the natural resources at the core of colonialism over many centuries. As we say in the editorial, data extraction by the Global North from the Global South “creates new human ‘data relations’ and a new social order; even social life” – and this poses the risk that the Global North can control data flows and tilt not only the data landscape but the entire digital economy.
6
AI work can lead to greater inequality and increased marginalisation
We have heard the argument that workers in the Global South benefit monetarily through AI-related work. We nevertheless suggest there is an imbalance because the short-term work (often in poorly paid and precarious work) can, over the long run, lead to greater inequality and further marginalisation. We conclude that academics and society at large need new ways of understanding and managing these risks.
7
AI policy makers need to be flexible
Policymakers who seek to create AI legislation need to account for its fast-moving fluidity alongside the digital maturity in individual countries and regions. These policies should also reflect the rights and ethics of those doing the data work and helping to develop these new algorithms and AI technologies, particularly those in the Global South who risk further marginalisation.
8
Policymakers should define risk, evolve responses and consider data protection
Our editorial has 3 broad conclusions:
- first, policymakers must define the key risks from AI while simultaneously demystifying less severe risks within a broader context that may include benefits as well
- second, policy needs to respond to the evolving situation and resist becoming static
- third we need to revise current policies on data protection while at the same time holding AI technologies to account within the existing framework
9
AI policymaking requires multidisciplinary collaboration
We advise to be on guard that people and organisations not rely on their own professional judgment as they lean on AI to make judgments and set core values. The paper also highlights the importance of multidisciplinary collaboration between important stakeholders, including innovators (academia or industry), policymakers, institutional organisations collecting the data and, importantly, the public.
The UK Prime Minister, Rishi Sunak, will host the AI Safety Summit 2023 on 1-2 November at historic Bletchley Park near London, bringing together governments, AI companies, civil society groups and other experts. They will examine the risks of AI and how they can be mitigated through internationally co-ordinated action.
Featured faculty
Michael Barrett
Professor of Information Systems & Innovation Studies
Featured research
Arora, A., Barrett, M., Lee, E., Oborn, E. and Prince, K. (2023) “Risk and the future of AI: algorithmic bias, data colonialism, and marginalization.” Information and Organization
Related content
The AI Safety Summit will be hosted on 1-2 November 2023 in Bletchley Park.