In today’s workplace, people often get feedback from peers, supervisors and other human beings as well as from machines including those driven by artificial intelligence. But what is the interaction between these 2 types of feedback in terms of how people learn from their failures?
This question is particularly important because of the rise of Generative AI in the workplace, as it stresses the role AI could play not only in the production of knowledge or tasks, but also in getting humans to learn and improve over time.
A new study co-authored by Professor Thomas Roulet of Cambridge Judge Business School, together with Tengjian Zou (Zhejiang University, China) and Gokhan Ertug (University of Adelaide, Australia), finds that machine feedback acts as a ‘catalyser’ to motivate individuals to learn more from failure feedback given by other individuals – so this interplay can be a key element to improving performance and outcomes.
Two ways AI failure feedback boosts people’s learning
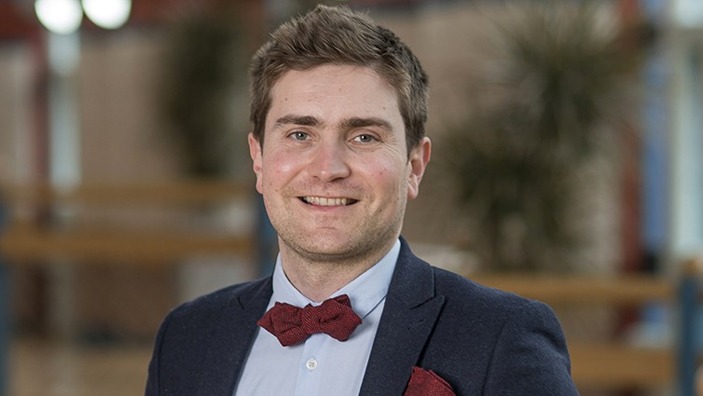
“Machine failure feedback facilitates learning both directly – by leading individuals to learn from their failures – and indirectly – by amplifying the learning effect of failure feedback provided by other humans,” says the study published in the Journal of Business Research.
The study is based on a dataset of 1.5 million observations from more than 93,000 participants in an online programming contest, which allows learning outcomes to be “measured precisely and objectively” because contestants’ codes are locked in and then evaluated by machine.
“Previous research on learning from failure has mostly focused on how people learn from failure feedback provided by other people, be it their supervisors or peers,” says study co-author Thomas Roulet, Professor of Organisational Sociology and Leadership at Cambridge Judge. “We have had little understanding of how people learn from failure due to machine feedback, nor the interaction between the 2 types of feedback – so our new study helps to fill this important gap.
“We will increasingly receive automated feedback from AI in the workplace – we already do so for our spelling, and will soon for many other more complex and knowledge-intensive tasks we do in our everyday jobs.
Key finding is that people inherently trust feedback from machines
“A key aspect of this study is that the machines used are designed to catch nearly every possible error that a contestant may have in a code (as AI would do when assessing work tasks), so contestants inherently trust the failure feedback from the machines,” Thomas adds. “The study finds that such purely objective evaluation of failure provided by machine, whether they are GenAI or more simple software, can help someone learn better from failure based on human evaluation as well, so that’s an important finding.”
So what is failure? The study broadly defines failure as “performance that falls short of a desired outcome” – providing examples such as an employee’s ideas being rejected by a company’s evaluation committee, an unsuccessful cardiac procedure performed by a doctor, a project not living up to expectations, or a new enterprise that doesn’t succeed.
Thomas co-authored a review in 2018 (see yellow box below) that examined the challenges associated with learning from failure: this is usually harmful to one’s self-esteem, as people tend to naturally suppress failures from their memories and learning processes. Yet failures provide a huge opportunity to learn, because they contain more information for more radical improvement compared to success.
“Learning from failure is a process by which individuals reflect on what might have gone wrong in their practices and develop solutions to avoid similar mistakes in the future, ultimately improving performance,” the new study says, adding that motivation to learn from failure is a key determinant in whether such failures are prevented in the future.
“Prior studies have examined how people learn from their failures,” authors of the new study continue. “In many cases, failure is revealed to individuals by feedback from external parties” including peers, as “failure feedback from them can make failures visible to a focal actor and trigger learning. This visibility raises the focal individual’s awareness that some of his or her practices might be problematic. As a result, this individual will be motivated to learn from these failures, for example by taking actions to scrutinise the failure information, figuring out the cause of failures, and remedying problematic practices.”
For example, a field experiment “showed that the performance of soldiers improved when they were debriefed on their failures after each training day”.
Why machine feedback can motivate people to learn from colleagues
The study finds that machine feedback on failure motivates individuals to learn from failure feedback provided by their peers.
The study finds that machine feedback on failure motivates individuals to learn from failure feedback provided by their peers – so the more people get failure feedback from machines translates into more likelihood that people are aware of the potential to also learn from peer feedback and to allocate resources to learn from such peer feedback. Motivation to learn from failure is one of the key levers, in addition to opportunities to learn (how failure can be analysed to improve performance) and ability to learn (the skills related to analysing and capitalising on failure to progress).
“In summary, we argue that machine failure feedback raises individuals’ awareness of the potential to learn in general. This motivates individuals to allocate resources to learn more from peer failure feedback as well,” the authors say.
The research finds further that the degree to which machine failure feedback improves learning from peer failure feedback (the “catalysing effect”) depends on how closely related the tasks are on which the feedback is provided by machine and humans.
Study based on data collected from machine evaluated programming contests
The study is based on data collected from Codeforces, which is an online community that hosts programming contests for people (students and professionals alike) to improve their coding skills; the study focuses on 988 contests (each averaging nearly 5 hours) between the launch of Codeforces in 2010 and 2018.
In these contests, participants enter a coding problem solution and this is automatically evaluated by an automated machine. If the entered solution does not solve the problem, the machine indicates that there are errors without specifying the solution, so contestants need to figure out what they did wrong and correct those errors – in other words, learn from their failures. In addition to this machine feedback, contestants also receive failure feedback from other contestants who can view and identify fellow contestants’ coding errors.
Contestants receive points both by solving their own coding problem and by successfully correcting another contestant’s solution – which in contest lingo is known as a successful ‘hack’ of another’s solution. Performance is then measured by a contestant’s relative performance compared to others in that particular contest.
Why organisations should provide both machine and human feedback
The machine element may help address built-in biases through human-to-human learning.
The authors say that practical implications of the research suggest that organisations should provide both machine and human feedback to employees. The machine element may help address built-in biases through human-to-human learning. In the era of GenAI, simple feedback mechanisms provided by AI that would have learnt the nature and process of a specific task, and ways to improve performance, could be crucial in complementing humans and getting them to sharpen their skills and develop new ones.
“For example, as part of their annual performance feedback, organisations can implement an information system to provide performance feedback to employees based on rich data, such as sales, project progress, and number of patents filed, in addition to the currently common practice of providing performance feedback from employees’ supervisors. For complete tasks such as writing a report, analysing data, producing a presentation, AIs could provide direct suggestions of improvement which would be more likely to be taken on board compared to human feedback, and would also generate more interest for human feedback.”
The study suggests some topics for future research in this area. The machines in the study identify only one type of failure feedback – whether there are coding errors – so machines could also be designed to provide other types of feedback as well. This could provide insight as to whether multiple types of feedback enhance the learning effect of each other to amplify overall learning, or whether this might “distract” individuals from each mistake and diminish overall learning.
The ways to harness the power of AI in the workplace are still evolving
Thomas adds: “We are only at the start of discovering how AI can be harnessed in the workplace, and in an era of fast-paced change we need more than ever to help employees reskill and develop their existing knowledge and approaches to work tasks.”
In addition, the authors suggest that there could be research on how machine feedback and human feedback interact when such feedback is about success rather than failure.
An earlier review article by Thomas Roulet identified 3 mechanisms of learning from failure
The 2018 review co-authored by Thomas Roulet in Academy of Management Annals, which analysed previous studies on learning from failure, identified 3 mechanisms through which individuals, groups and organisations learn from failure. Here are excerpts regarding each mechanism:
1. Opportunity to learn from failure
“Opportunity to learn refers to the scope of information and the time that allows actors to learn from failure events. Information-based opportunity refers to the amount of information that is available about similar failure events because such events can provide information about failure causes. Time-based opportunity refers to the time that is given to actors to reflect upon failure events and to analyse the information that can be derived from the events to learn from them and the time in which to execute an action that is related to a failure learning activity. Information-based opportunities are usually studied by quantifying the amount of available information about similar failure events (number, frequency, recency), information access owing to group composition, organisational members’ networks and information diffusion inside or between organisations.”
2. Motivation to learn from failure
“Motivation is the desire or willingness to act in a certain way and, in the context of error and failure learning, the desire or willingness to invest in reducing adverse event frequency. Motivation to learn from failure therefore refers to the resource levels that are devoted by individuals and organisations to failure learning activity; such resources include attention and operational investments. Effective learning processes require individuals and organisations to allocate cognitive resources to (i) correctly identify and analyse error and failure causes and (ii) search for and implement solutions that prevent similar errors or failures in the future.”
3. Ability to learn from failure
“Ability to learn from failure concerns the capacity to identify and report failure; understanding failure leads to finding and implementing solutions to prevent future failures. Individual and group level studies are concerned with training, emotional responses, shared goals and managerial style, as well as the interaction between ability and motivation. Organisation-level studies rarely measure directly ability but rather conclude that the unobservable variances across units or organisations are due to differences in ability. In healthcare, where geography makes competition between hospitals less of an issue, checklists are used for the transfer of best practices across units, which raises the ability to learn in organisations.”
Conclusion of 2018 review article
“An organisation that seeks to enhance error and failure learning should analyse the causes of its most common errors and failures. To maximise the opportunity to learn, the organisation should not only study its own but also the events of similar organisations. Further, including near-misses would add information and suggestions for ways to avoid an
adverse outcome after a process error has occurred. When attributing causes, management must ensure that operators are not unduly blamed and if the cause is found to be operator error, look for systematic such errors to find if there are structural or organisational factors leading to operator error.”
Featured research
Zou, T., Ertug, G. and Roulet, T. (2023) “Learning from machines: how negative feedback from machines improves learning between humans.” Journal of Business Research
Dahlin, K.B., Chuang, Y.-T. and Roulet, T.J. (2017) “Opportunity, motivation, and ability to learn from failures and errors: review, synthesis, and ways to move forward.” Academy of Management Annals