Five important challenges in dealing with the coronavirus crisis can be addressed through machine learning and artificial intelligence, says article co-authored by Professor Stefan Scholtes of Cambridge Judge.
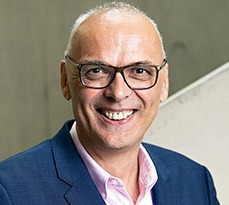
Five of the most important challenges in responding to the coronavirus crisis can be addressed through recent developments in machine learning (ML) and artificial intelligence (AI), says an article in the journal Machine Learning authored by a team of academics at the Cambridge Centre for AI in Medicine, including Stefan Scholtes, Dennis Gillings Professor of Health Management at Cambridge Judge Business School.
The integration of these ML and AI techniques into local, national and international healthcare systems will save lives by better utilising scarce healthcare resources and providing personalised patient management plans, the article says.
The five areas highlighted in which ML and AI can play an important role are:
- Identifying people at the highest risk of being infected by COVID-19, by integrating electronic health record data with a “multitude of ‘big data’ pertaining to human-to-human interactions” – from sources such as cellular operators, airlines and social media. Through such techniques, “we can provide more accurate patient risk scores that will help clinical professionals decide who needs urgent treatment (and resources), and when.”
- Helping to determine the most efficient treatment for each patient based on data from other patients including treatments administered, which is important given that coronavirus symptoms and disease evolution vary widely from patient to patient. “We can use machine learning to answer key ‘what if’ questions about each patient, such as ‘What if we postpone a couple hours before putting them on a ventilator?’ or ‘Would the outcome for this patient be better if we switched them from supportive care to an experimental treatment earlier?’”
- Providing insight into the differences between policy approaches, which work better, and how to design and adopt improved policies to ensure that routine decisions are made in a more coordinated and timely way. “Coordinating experiences across different actors allows us to more systematically quantify policies and their efficacies, thereby informing the design of improvements.”
- Recruiting subjects for randomised clinical trials from identifiable subgroups, and assigning them to treatment or control groups in a way that speeds up trials. “These methods have been shown to significantly reduce error and achieve a prescribed level of confidence in findings, while also requiring fewer subjects.”
- Helping to quantify the degree of uncertainty in our knowledge of the disease, given the very limited experience and limited data surrounding COVID-19. For example, ML can help quantify uncertainty on predictions of patient-level outcomes in order to guide hospitalisation and therapeutic decisions.
The article then goes on to discuss three techniques to implement the variety of AI-based models discussed, using the UK as an example.
- Individual-level interface: Based on individual risk assessments issued by AI models, patients can be alerted to undergo testing via text, email or mobile phone calls, and individuals in high-risk areas where a significant number of cases have been diagnosed can be notified.
- Hospital-level interface: In-hospital infrastructure can be complemented with risk-modeling applications that alert clinicians to the evolving risks of patients within each hospital.
- Nation-level interface: Centralised applications can continuously update officials with the latest epidemiological data on COVID-19, and this can be directly linked to the NHS patient registration database.
The article – entitled “How artificial intelligence and machine learning can help healthcare systems respond to COVID-19” – is co-authored by Mihaela van der Schaar, Ahmed M. Alaa, Andres Floto, Alexander Gimson, Stefan Scholtes, Angela Wood, Eoin McKinney, Daniel Jarrett, Pietro Lio and Ari Ercole of the Cambridge Centre for AI in Medicine.